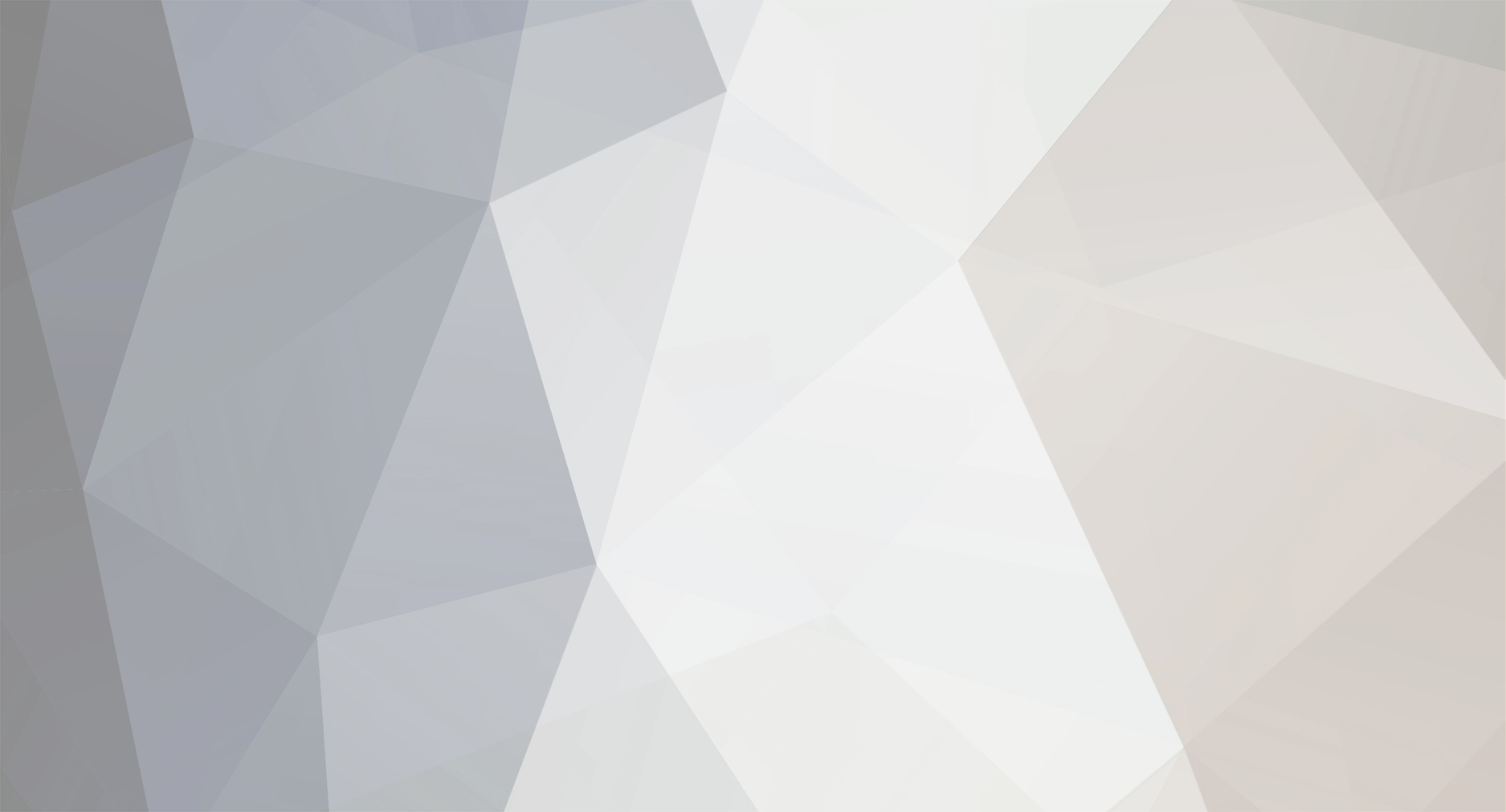
qpoiz
Members-
Posts
9 -
Joined
-
Last visited
Recent Profile Visitors
The recent visitors block is disabled and is not being shown to other users.
qpoiz's Achievements
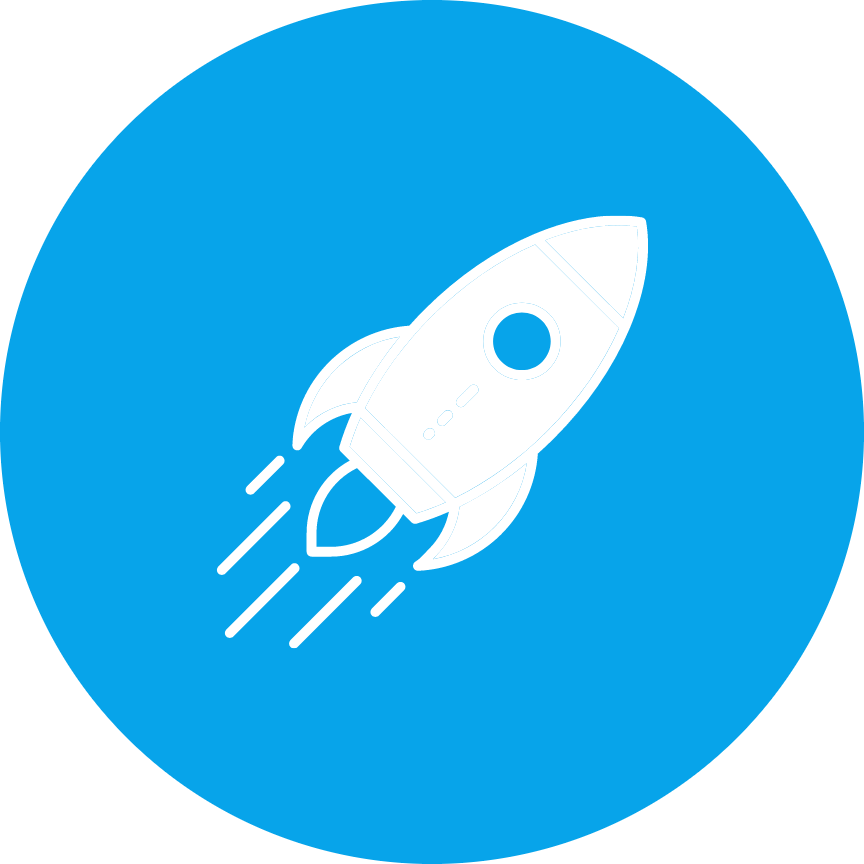
Decaf (2/10)
-1
Reputation
-
I received a BS in biochemistry in 2014 and took a gap year to knock out basic math/CS/physics courses. The goal was to specialize in ML/statistics to get involved in AI but have realized going to school to learn hardware (over software) would be more valuable long term. That being said, I've looked into BSEE curricula to get a general idea of the work ahead me, and it looks like I will need to crank out at least 40 credits of EE courses (10 courses) to be competitive for a MS/PhD. Logistically it seems too little, too late for me at this point to get an EE MS/PhD, but I was hoping there may still be solutions that I am not aware of to reconsider. I would love to hear your experience. Thank you for your help. EDIT: I completed calc 1-3, linear alg, diff eq, combinatorics, statistics, physics 1/2, data structure, algorithm: 4.0 postbac, 3.3 bac. I can knock out 5 freshman/sophomore EE/machine learning courses this summer.
-
qpoiz reacted to a post in a topic: Debating biostatistics vs computational math
-
qpoiz reacted to a post in a topic: Choosing a discipline
-
qpoiz reacted to a post in a topic: Choosing a discipline
-
qpoiz reacted to a post in a topic: Choosing a discipline
-
qpoiz reacted to a post in a topic: Choosing a discipline
-
@compscian, that is very admirable and awesome to hear that you successfully switched fields. If you took 6 ML courses (what courses specifically if I may ask), I think the barrier to entry for me would be higher. And I may not have the money/time to do additional post-bac work (although CMU/UWashington seem to have affordable non-degree tracks). What is your opinion on human-computer interaction programs (CMU, UMich, Stanford)? At first glance it seems to have a lesser emphasis on ML/AI but it seems much more accommodating to non-CS degree holders and it may serve as a good point to horizontally transition into ML dept. UW does seem like it has a really great interdesciplinary approach between EE/stat/CS. I also saw that CMU actually offers a joint PhD in statistics and machine learning and Princeton looks to do something similar in the near future. What do you think was the biggest factor for your success/admission? I'm assuming you applied only to top programs (Stanford, UT Austin, Columbia, Berkeley, Michigan) as well. EDIT: It also seems that going into PhD statistics could be more viable/realistic as the pre-req is not as steep as that of EECS (like UChicago) and take a more mathematical (rather than CS) approach to machine learning (anecdote). It's said that a CS program is less rigourous on hard math training but I'm curious if students have the opportunity (in UWashington, for example) to take advance pure math courses like algebraic geometry.
- 7 replies
-
- applied mathematics
- biostatistics
- (and 5 more)
-
@compscian, so I had thought that EECS was simply a notation difference from EE and CS programs but it seems that there is a difference in curriculum. I had considered doing an MS first as well but I thought that it would be more cost effective (albeit more risky) to take a few classes (that are part of the core PhD curriculum) as a non-degree while working in a lab. I would then apply the following cycle. I actually looked into Stanford, Caltech as well, but Caltech CMS did not have a MS option. MIT also has a similar program called CDO that looked promising. May I ask how you transitioned from MechE to ML/AI - did you take classes as a post-bac? If so how much additional coursework/time did you take to be competitive? Do you think the best point of contact to inquire about a dept's emphasis on EECS would be the program director or professors within the dept? I'm trying to transition into a diff. field late (26 yr) and essentially navigating through this by myself. You've been a tremendous resource, @compscian.
- 7 replies
-
- applied mathematics
- biostatistics
- (and 5 more)
-
abstract_art reacted to a post in a topic: Choosing a discipline
-
Thank you very much for your input. I am curious if, considering my background in biochemistry, getting into a PhD EECS program will be plausible. Also are you familiar with computational science/engineering (Harvard, UT Austin)? At first glance it seems similar to applied math in that it focuses on application research but its a "nascent" field that commonly accepts people with life sciences background and may also study ML/AI from an interdesciplinary approach . Lastly how much statistical training could I get by pursuing EECS? I know this varies by school but was curious to know generally what I should expect. Thank you again for your comment. It has been tremendously insightful.
- 7 replies
-
- applied mathematics
- biostatistics
- (and 5 more)
-
I am interested in continuing my education in math and I know that I'd eventually like to work on brain-computer interface (theory and application) like mind uploading but was curious if there is a discipline that merges computational neuroscience, biostatistics, AI, and cybersecurity: providing a rigourous curriculum that can be used to pursue these fields. Any input would be greatly appreciated! This is ultimately to maximize my chances of being employed, having a successful career long term. If the opportunity exists, I would equally like to learn more about AI (neural net) and cybersecurity, and I currently enjoy the statistical, predictive modeling (machine learning) work that I do in genetics (similar to data science). I have thoroughly looked through gradcafe, stackexchange, quora, reddit and amassed math topics important in each field. I have highlighted common topics and would like to get you guys' input on the accuracy of this list. MATH TOPICS FOR EACH FIELD cybersecurity - applied number theory (abstract algebra), combinatorics (graph theory), algebraic geometry, information theory, asymptotic analysis, finite fields computational neuroscience - information theory, systems theory (nonlinear dynamics, dynamical systems), evolutionary algorithms (Monte Carlo), state space analysis, signal processing, probability theory AI/ML - neural networks, genetic algorithms, information geometry (Riemannian geometry, information theory, Fisher information), algebraic geometry, manifold geometry, learning theory (Fourier analysis), probability theory, game theory (topology, measure theory), graph theory, Model Free Methods RECOMMENDATIONS Some have recommended biostatistics programs because the curriculum offers a fair amount of 'theoretical' math work. Others, however, have said that biostatistics is a bad choice - sticking to CS or EE would be better. There is always the option to go into pure math but I am concerned about employability of a pure math PhD compared to an applied math PhD. I have played with the idea of work towards becoming a fellow of actuarial science simultaneously instead to gain statistical training - although this would be more oriented towards business, not science There is also the fact that I have a BS in biochemistry. I have done post-bacc work for CS fundamentals, calculus series, diff. eq., linear algebra, statistics, combinatorics, but there is a legitimate chance that I may not have sufficient background for fields (like statistics or applied math) other than biostatistics. I have looked heavily into degrees for applied/computational mathematics, scientific computing (UPENN, Rice, JHU, MIT, Stanford, Maryland) but it seems that these fields are more broadly focused on application reseach for physics, chemistry, biology (like engineering). I've also looked into mathematical biology (aka biomathematics) but it seems not a lot of schools have such a department - it's commonly housed under computational/systems biology. Thank you very much for your time and help!
- 7 replies
-
- applied mathematics
- biostatistics
- (and 5 more)
-
Debating biostatistics vs computational math
qpoiz replied to qpoiz's topic in Mathematics and Statistics
Thank you, @localfdr. Yes, you are correct that I was using the wrong terminology and could've explained better. Essentially I would like to study what is traditionally known as math in an applied field and also learn statistics. Would you say that pursuing a statistics phd is better than a biostatistics phd for my intentions? Is statistics phd better because most biostatistics do not offer theoretical math courses as elective? Considering that I am not from a math background and I have a relatively short record of math, how difficult would it be to gain admission into an applied math and statistics phd program? Thank you again for your input. -
Hello gradcafe, I am interested in pursuing a PhD in biostatistics but am concerned that I may not get to work with theoretical math. Ideally I would like to apply theoretical math in fields like computational neuroscience, AI, cyber security, crypto-currency and I presume that these disciplines are degrees apart from biostatistics. On the other hand, I very much enjoy (big data) analytics, predictive modeling that comes with studying biostatistics. From you guys' experience, is there a degree/field that has a rigorous curriculum for both biostatistics and pure math? I would most likely take programming/machine learning courses as electives. There is also the (anecdotal) consensus - learning applied math (like stat) is more marketable than learning pure math - that adds to my dilemma. Should I focus more on degrees like computational science and engineering; computational and applied mathematics and become a fellow of actuarial science (FSA) on the side? Although this teaches statistics, I cannot say how related it will be to biostatistics. I have a BS in biochemistry so getting the prereqs lined for a PhD in pure math will be much more difficult. I did my post-bac to take calc 1-3 sequence, linear algebra, diff. equation, real analysis, statistics, and combinatorics. Thank you very much for your time and help.
-
What is your opinion on the backdoor (non-degree) method?
qpoiz replied to qpoiz's topic in Applications
Thank you for your reply, TaeruK. Going back to your comment on students not succeeding, was it because they couldn't handle the workload? Did they struggle to learn the material quickly or maybe did not expect what being a graduate student meant? I think you bring up a good point. The question then becomes, how easily could I work in a biostatistics lab as a non-degree student. Ideally I'd like to work under the PI who will advise me, but considering that my research interests may change and, again, depending on how easy it'll be to work in lab, this may be very challenging. -
I was curious if some of you guys tried entering as a non-degree student to gain admission into the PhD program the following academic year. Specifically, I would receive a non-traditional graduate student status to take graduate-level courses, which are transferable and part of the PhD core curriculum. Potentially, I would work as a part-time school employee to possibly receive tuition assistance. The benefit is that I would not be tied to the full-time student tuition level, and considering that Masters program funding is scarce, this method may be more economical. I would only pay based on # of credits taken. But the obvious downsides are that admission is not guaranteed and there is no internal funding. Also I plan to work as a school employee to receive tuition exemption. For public schools (e.g., U. of Washington and UCLA), tuition assistance is very generous. Just in case, are there funding opportunities for prospective "grad" students like me that I'm not aware of? Although not guaranteed, is admission likely going this route considering that I would have formed relationships with the dept. faculty and staff? Any thoughts, inputs, tips will be greatly appreciated. Thank you very much for your time and help. Edit: I have a decent (3.3) uGPA. I received a BS in biochemistry at a top 25 school but wanted to specialize into a more quantitative field (biostatistics). I took time off to take 11 undergraduate quantitative courses in the summer and fall semesters and was successful (4.0 GPA). I have research experience, publication, all the necessary documents but was involved in a car accident that prevented me from writing the GRE before application deadlines. Before considering non-degree programs, I was going to apply to the MS program first instead of the PhD program because I felt that my quantitative background may be lacking and worried that my age (27 yrs) will put me at a disadvantage against other PhD applicants.