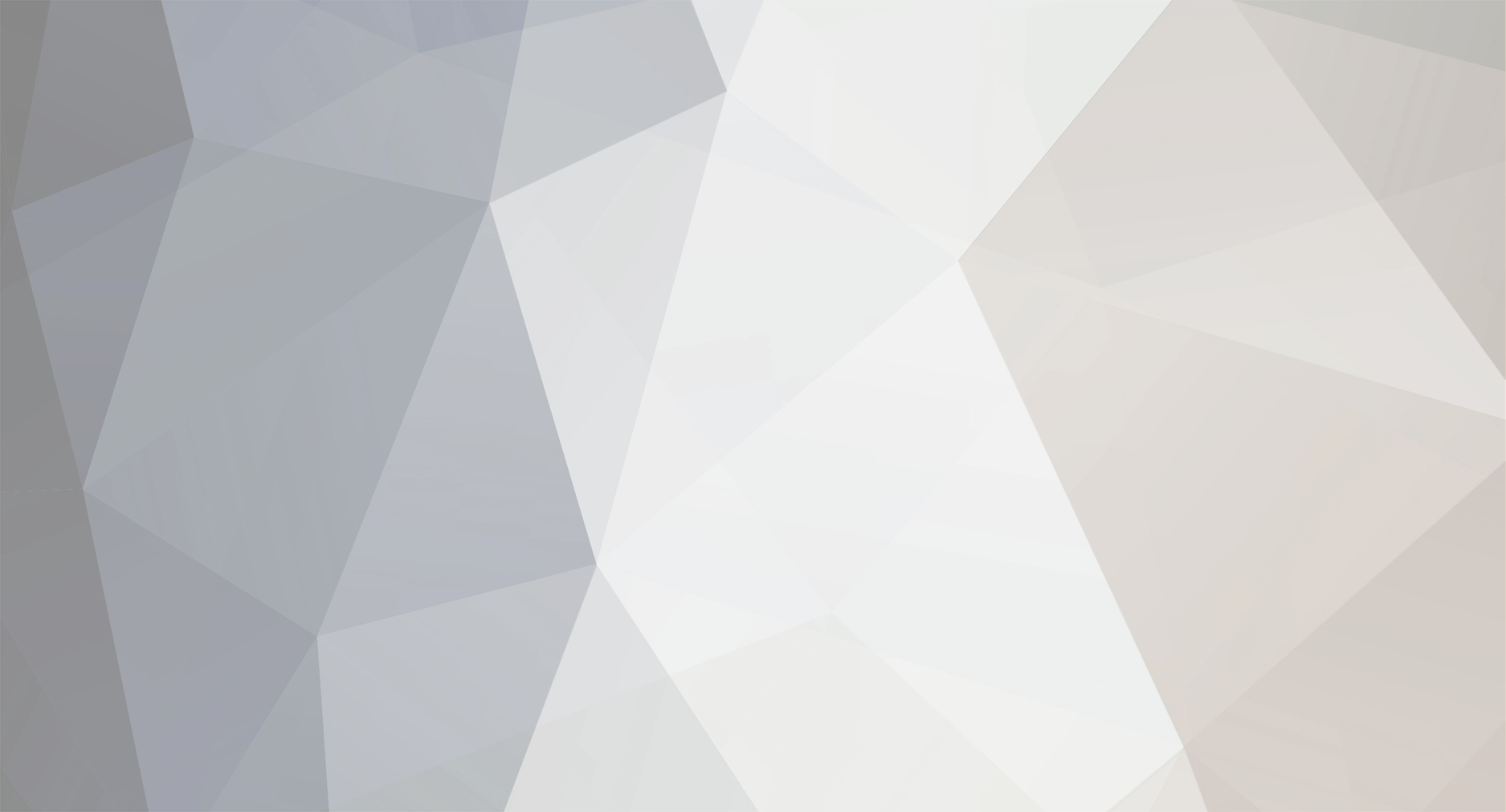
Dawnbreaker
Members-
Posts
40 -
Joined
-
Last visited
Profile Information
-
Gender
Not Telling
-
Application Season
2015 Fall
-
Program
Applied Mathematics
Recent Profile Visitors
2,277 profile views
Dawnbreaker's Achievements
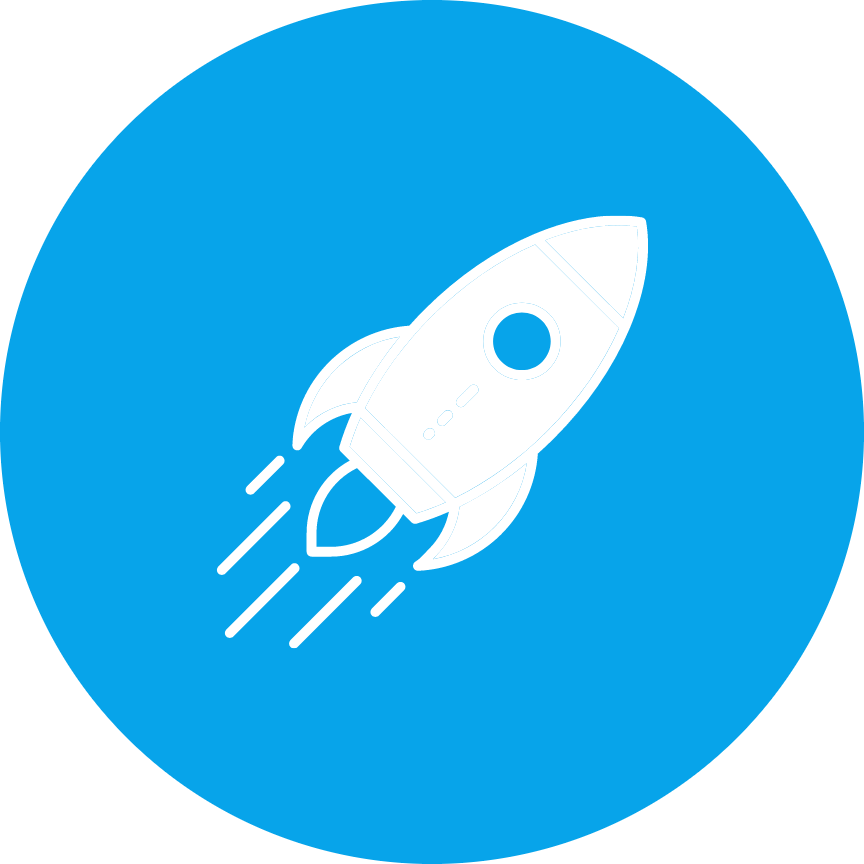
Caffeinated (3/10)
7
Reputation
-
liyu reacted to a post in a topic: PhD in Operations Research: Cornell ORIE v.s. Columbia IEOR v.s. Berkeley IEOR
-
uiop reacted to a post in a topic: PhD in Operations Research: Cornell ORIE v.s. Columbia IEOR v.s. Berkeley IEOR
-
A couple years back, I ended up visiting both programs. I was particularly unhappy with Berkeley IEOR, for the following reasons: 1. Berkeley is vert strong in Stats, EECS, and Math. All the star faculty are in those departments and not IEOR. If you want to do theory, you'll be competing with students in CS theory, which will be a very hard fight. Similarly Statistics and Math departments have great students doing optimization and probability theory. Thus, there is absolutely no reason for someone to choose IEOR over Stats, CS, or Math; and thus all students there seemed to have an inferiority complex. 2. As for the applications, again IEOR doesn't seem to be doing anything very impressive. The west coast scene is mostly driven by the software industry, and focus primarily on CS and Statistics. Bottom line, the IEOR dept of Berkeley is heavily overshadowed by the other departments there. I am not aware of any students in IEOR who work with outside faculty, and if they do, chances are that they will switch programs. Given these factors, I would advise against Berkeley IEOR, especially given that you have an offer from Columbia which arguably has a stronger IEOR department, and would likely provide better moral support.
- 11 replies
-
- columbia ieor
- cornell orie
-
(and 1 more)
Tagged with:
-
Range of opportunities with a PhD is much much more than range of opportunities with an MS. If you really want to do the exciting work that is hyped up in big data, you need to be the head of a team with 3-4 people working under you (the MS in DS type people). For that you need a PhD. I perceive MS in data science as too short sighted. An MS in data science over specializes on the type of skills that are currently in demand in industry. If the demands or methods change, much of the DS curriculum is obsolete. An MS in CS or Statistics on the other hand provides the necessary foundations, in addition to skills of value in industry. When applying for a PhD program, I am pretty certain that strong departments would prefer an MS in CS or MS in Statistics. If you go the MS in DS route, you might be forced to do a PhD in data science with some umbrella program as your home unit (similar to Stanford where ICME is not a department). This is far from ideal if you decide to go the academia route or even top industrial labs. I perceive interdisciplinary and data science programs as weaker compared to an established department. Given a choice between MS in CS, MS in Statistics, and MS in DS: nearly everyone will choose the former two. Hence, your cohort is going to be weaker. I'll pick UCLA for all the above reasons. In addition, I'd take at least 3-4 CS courses (data structures & algorithms, software development, graph theory, and an applied ML course like vision or speech processing). It'd be ideal if you can get an MS in CS along the way too!
- 4 replies
-
- phd
- statistics
-
(and 3 more)
Tagged with:
-
Dawnbreaker reacted to a post in a topic: What is better - Berkeley or Caltech
-
@myh Hi.. among these 4 (and in general too), Berkeley and Stanford have the strongest programs. Based on my interaction with students from both programs, I have observed that Stanford's program tends to be more slanted towards CS. If you observe, quite a few Stanford MS&E professors have PhD in CS. I felt that many students at Stanford were unhappy because they perceive themselves as being second fiddle to CS - they try to do work that appeals to a CS audience, but obviously they cannot be as good as a CS-proper program. Berkeley on the other hand is a bit more theoretically inclined. They primarily stick to optimization, but also foray into "newer" and applied areas like data mining, network flows, power grids, robotics etc. I feel that these projects are oriented towards both general OR/CS as well as domain specific audience. I think they try to see how an OR perspective adds to a problem in data mining or robotics, as opposed to doing low quality work in data mining or robotics. So you'll see a lot more collaboration, and multiple PI names on the paper. I think this is a good middle ground to retain the theoretical rigor of OR, but at the same time get exposure to newer and "hotter" research areas. It is true that Berkeley on the whole, and IEOR in particular had a funding crunch some years (3-4) back. However, they have recovered gracefully. I believe they have given fellowship offers to all students this year (a college told me), and 100% of students have got full funding for the past 3 years or so. They even got a part of new building (CITRIS) and renovated their main building. You shouldn't be worried about funding, but do make sure with the program manager. I am only familiar with Peter Frazier at Cornell who does interesting work. However, both Berkeley and Stanford can offer more in nearly every metric than what Cornell can offer you. I'd recommend that you choose one of these two. If you want to do more applied work at interface of CS, Stanford is likely a better option. If you want to do more mathematically grounded work, have a shot at faculty positions, but also do some applied projects from time to time, Berkeley is the best option.
-
I don't think this is accurate, and it doesn't even agree with your last line about Aaron Clauset's findings! Let me clarify, I don't believe in rankings as a strict indicator (rank 6 not necessarily better than rank 7). However, there are definite tiers. Implicitly, you are creating a tier yourself with something like "top 10". Why did you come up with this magical number 10, and not something else like 15 or 20? IMHO, it's pretty clear that top 10 is not homogeneous by any means. For example, in EE; MIT, Stan, UCB, Caltech, and (possibly) UIUC clearly break from the rest. Head to head, MIT will definitely win much more students than Michigan for example. The causation is not high rankings, but rather the understanding that MIT has a higher standard of research, which in turn reinforce the rankings. To say that difference in rankings is negligible is too much of a stretch. In CS, the difference between the top 4 or 5 (MIT, Stan, UCB, CMU, UW) and the rest of top 10 is even more stark. No one with an offer from one of these programs is going to choose something else, unless there are other factors at play like two-body. They also produce much more faculty. I however agree with you on funding. I wouldn't do a PhD without guaranteed funding. But, I am pretty sure a top school like Berkeley wouldn't admit anyone without funding. I think the OP is just waiting for confirmation on fellowship, which if he doesn't get, should get a TA/RA offer (the same happened with me). With regards to NRC rankings, they are outdated.
-
bandinterwebs reacted to a post in a topic: How Much Do You Weigh Ranking?
-
@bandinterwebs Also look at people from Haas, some people there also seem to do relevant work.. Anyway, to answer the opening question, I personally think the ranking difference in this case is quite significant. I'd highly recommend Berkeley. Best wishes for your decisions and grad school experience
-
Hi @bandinterwebs.. As my signature suggests, I'll be joining MechE. However, my research interests are much more on the optimization, control, and statistics end of things. I'm actually trying to see if I can get one of Profs. Goldberg or Lavaei as my adviser or co-adviser. I got admitted for Fall 2015, and deferred admission. So had a ton of time to do groundwork on various programs. I am not 100% sure about production and logistics. How fixed are your interests? I mean, Berkeley did admit you, so there must be some research interest overlap! Check out Rhonda Righter and Lee Flemming. They seem to be doing related work. Btw, have they already approved your fellowship, or are you on some sort of wait-list? I assume you'll get TA/RA if you don't get the fellowship. Cost of living is certainly a concern, but definitely manageable with a little compromise and wise spending.
-
@bandinterwebs To be honest, Berkeley and Virginia Tech IMHO is a no contest. Berkeley is far superior in terms of reputation - both in academia and industry. It's also a great time for optimization and data science in general, and specifically in the Bay Area. The only reason to not choose Berkeley is if your research is very focused on particular areas like Supply Chains or Production/Manufacturing. However, as said before analytics, data science, and related problems are all the rage these days. I'm personally very familiar with the work of many OR faculty at Berkeley. Specifically, Javad Lavaei is a superstar in applying convex relaxation methods for distributed problems. Atamturk, Hochbaum, and El Ghaoui are also world leaders in various aspects of optimization and statistical decision theory. Ken Goldberg is of course a very famous Roboticist - with very close ties (multiple PhD students) to EECS and MechE programs. He uses learning and optimization for motion planning, robotic surgery etc - very interesting work. It's also worth noting that OR is very close to both the Statistics and EECS departments. Most end up getting an additional MS (with thesis) in either one of them depending on their inclinations. (OR+Statistics) and (OR+CS) are mouthwatering combinations for many job searches. So if you want to go the Industry route, Berkeley will definitely offer more - due to both program structure and location. I think summer internships are encouraged, and most do at least 2-3 summers over their 4-5 year stay in the program. It's also really strong for academia - they placed a recent grad at MIT. However, the only concern might be the high cost of living and financial situation of IEOR dept and UC Berkeley in general. I believe some 3-4 years ago, IEOR had a funding crunch. I think they have recovered well, but you should check with students and professors. How much are they paying you, and have they guaranteed full funding? PS: I realize that this sounds like a sales pitch, and honestly Berkeley doesn't need one. However, I am joining there and plan to work closely with many OR professors. Most of the above info are based on my own research and interaction with people there. As I said before, Berkeley and VTech is a no-contest. Berkeley and Stanford are the best places for new-age OR (intersection of optimization, statistics, and computing).
-
bandinterwebs reacted to a post in a topic: How Much Do You Weigh Ranking?
-
Hi @bandinterwebs.. In these cases, I think it would help a lot if you can identify the universities by name, instead of hiding them. That way, you'll get much better and directed responses. For example, my suggestions will be very different depending on what A is. If A is one of Berkeley or Stanford, I'd highly recommend that you go there. Though the programs are certainly theoretical in nature, the location, possibilities of summer internships, and overall culture will provide the necessary applied "flavor" you might need. On the other hand, I personally think that GATech and Northwestern are not as good as rankings suggest - they end up doing very theoretical work based on last century knowledge. I'd definitely pick a lower ranked program like UIUC or Cornell over them. PS: Note that I have a bias in my research interests towards statistical decision theory, stochastic control, and convex optimization. All of these are at the interface of IEOR, EECS, and Statistics.
-
bandinterwebs reacted to a post in a topic: Fall 2016 PhD Industrial Engineering - Need Advice on Studying Up Before School Starts
-
It might be helpful to mention your background, and what you already know. Much of OR is centered around the following, so it might help to brush up on these topics. More directed feedback is possible if you provide further info. Optimization - start with LP, duality etc. Also study convex optimization, numerical optimization (BFGS, conjugate gradient etc.). If time permits, get some exposure to integer programming using gomory cuts and other methods. Probability theory - basics of probability and statistics starting from common distributions and moment calculations. Bayes rule and its applications are also important. You should also study stochastic processess like markov process, martingales, and Gaussian process models (Bayesian framework) if time permits. Data structures and algorithms - do not under estimate the utility of algorithm design, complexity analysis, and programming in general. Some advanced topics like NP-completeness, approximation, and randomized algorithms are helpful too.
-
2016 ChemE PhD Applicant Profiles and Admission Results
Dawnbreaker replied to percheme's topic in Engineering
@deborah_caf Congrats! I'd say getting an admission in Dec for international students is indeed surprising and certainty early. Last year, my earliest admit was NU which was around Jan 10th. Wish you good luck for the other applications too (Where else have you apped? and which IIT are you from?) -
This is an extremely open ended question, and honestly I am not sure what to write. At the outset, I have a BS in systems and chemical engineering, and focused on optimization, data mining, and control (some process control, some networked control for smart cities). I think it is definitely possible to switch to a "more AI' field. (AI is a spectrum and not a single field) What I have observed is that AI is extremely broad. You can almost attach an "intelligent" prefix to anything which improves previous capabilities/results since no one really knows what intelligence is. That being said, one way to narrow down on interest is to look at AI and AI-related journals/conferences and identify some 2-3 venues which have publish most of the type of work you want to do. For me, the choices were ICRA/IROS, AAMAS, and CDC. For someone interested in vision, it could be CVPR, ECCV, NIPS etc. You can pick research communities like this, see which type of people publish regularly in such venues and apply to them. For example, AAMAS is mostly CS, ICRA/IROS is both CS and EE, CDC is mostly EE. So if these conferences interest you, a natural choice will be either CS or EE. Also, I think it might be a tad easier to get into EE programs than CS due to your background. If you are interested in hardcore CS areas of robotics/AI like vision, knowledge representation etc. the path is likely to be more difficult. On the other hand, if control, filtering, SLAM type of topics in robotics are your pick, you can get away with EE for which you stand a better chance.
-
Nallapar reacted to a post in a topic: Fall 2016 Chemical Engineering PhD
-
I accepted the offer from Caltech. However, due to some personal commitments that required me to stay in my home country (International student), I had to defer my offer to Fall 2016. I have been working as a research assistant in my undergrad university though, and also doing courses in consultation with Caltech professors. I think it has worked out great though - I can take courses and learn the material without any exam/grade pressure and do the research I like, with sufficient amount of free time. My research interest is at the interface of optimal control, machine learning, and economics. Applications are in energy markets, online auctions, clinical trials etc.
-
@ArgonI understand. Hope it works out to your satisfaction this year. Incidentally, what you said mirrors my impression from last year. My interests too didn't gel well with MIT and hence I applied to their masters program as a safety option. Funny how it turned out. Which type of computational work are you interested in - algorithm development for optimization, inference, control etc; or computation geared towards numerical simulation of ODE/PDE/stochastics? For the former, Wisconsin is clearly the winner in your list. For the latter, it's a good toss up between Minnesota, Berkeley, and maybe UIUC I guess. Congrats and best wishes
-
Argon reacted to a post in a topic: Fall 2016 Chemical Engineering PhD
-
Hi. If anyone has questions on Systems Engineering, in ChemE, EE, or ME, I can help with my 2 cents. Best wishes to all applicants. @Argon If you are comfortable with discussing, what happened at MIT? Faculty left, interested group is full, funding issues? Any idea where you are headed (UCB or Madison is a good option I'd guess).
-
- Assume no research This is a big negative. Top 5 PhD program across all fields expect good research if not publications. Also, good recommendation letters without doing research with them almost never happens. So heads up for that. -Strong interest in "mathy" fields within EE and CS, such as information theory, machine learning, data science, control systems. For these interests, I would rather apply to EE programs, or some sort of interdisciplinary EE and CS programs (like applied math). Info theory and control are nonexistent in CS. You can still do ML sitting in EE departments (in most cases directly, in a few cases indirectly through signal processing). Also from admissions point of view, EE is slightly easier than CS (statistically, there seem to be too many CS students for too few positions). So why not just do it in EE? You also have option of other "mathy" fields like communications, compressed sensing etc.