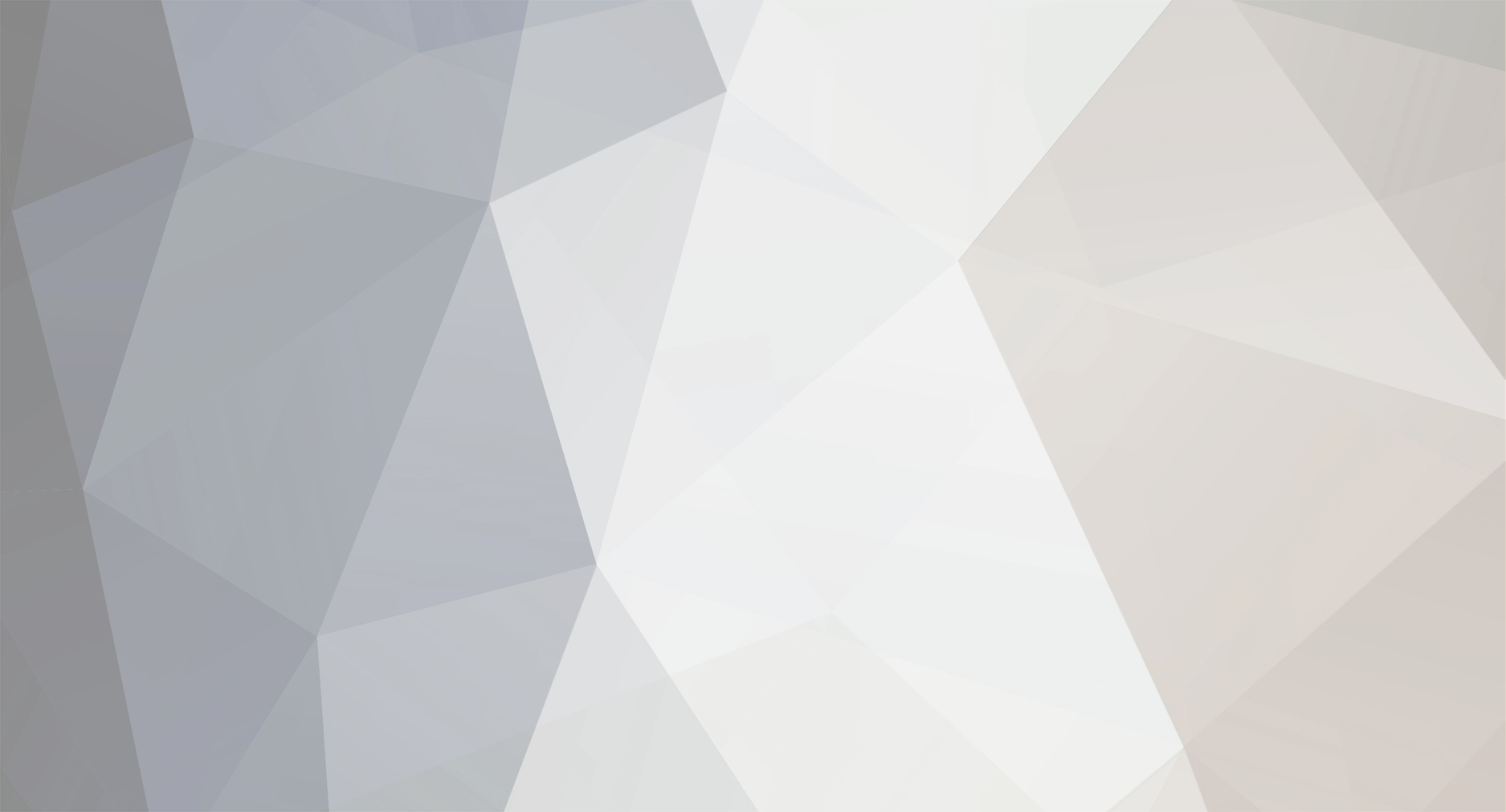
rfan
Members-
Posts
7 -
Joined
-
Last visited
Recent Profile Visitors
The recent visitors block is disabled and is not being shown to other users.
rfan's Achievements
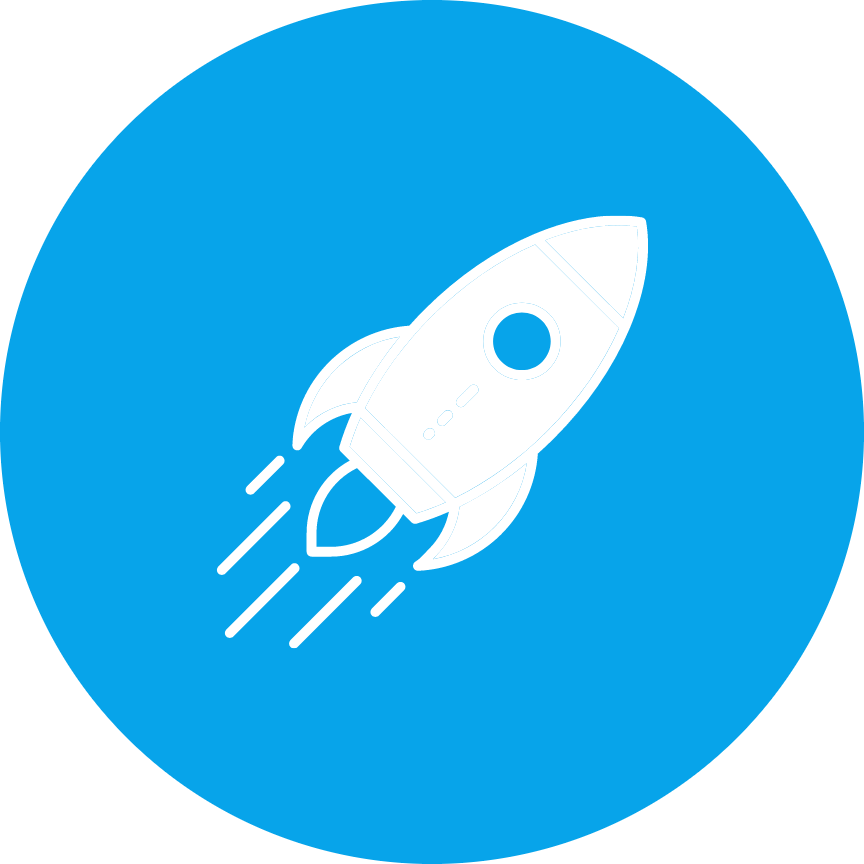
Decaf (2/10)
3
Reputation
-
To add on to this, I think it is likely that UNC and Colorado would allow you to master out if you decide you do not want to continue on to the PhD (can anyone with more knowledge of these programs confirm this?). So if you prefer think of either of these schools similarly to Michigan, I would say those might be better offers because you are guaranteed the PhD is you decide you want it but you don't have to worry about potentially applying for PhD programs again in a year in case it falls through at Michigan. I would say UNC and Michigan seem relatively comparable in terms of biostatistics programs (or really whole university in general). I would say UNC is a bit better rounded but Michigan is really great at genetics if that is what you like (UNC is no slouch but Michigan would be the best genetics outside of Washington). In general, I think these two schools fall in the 3-5 range for biostat departments with John Hopkins behind the top two (Harvard and Washington), but obviously personal preference comes into play here.
- 8 replies
-
- biostatistcs
- biostat
-
(and 3 more)
Tagged with:
-
To add to what I said above, I will say this about @bayessays comments above.... I originally worked in essentially data science (it wasn't really called that back then) with a masters in statistics. Most of the work is data science isn't far out of the reach of someone with a masters. The orientation is a bit different though, as being able to deploy production level code is often a more valuable skill than knowing some theoretical properties of a model. With that being said, moving up the ladder without a PhD can be somewhat hard, even if the tasks are still doable with a masters. Employers like seeing the PhD. It is why I went back for one, and I have zero regrets about it. It is especially true in any health related work. I am a data scientist in the healthcare industry, and it is quite hard to reach senior level positions without a PhD unless the work group sways more to software engineering (check out the data science team at RTI international as an example of this). For example, Duke has the Duke Clinical Research Institute, and they hire people for essentially data science roles, but it is challenging to do much without a PhD.
- 8 replies
-
- biostatistcs
- biostat
-
(and 3 more)
Tagged with:
-
rfan reacted to a post in a topic: Seeking Advice: UNC Biostat Phd vs. Colorado Biostat Phd vs. Michigan Biostat M.S. (all funded)
-
To the posters above, it seems like the person's admission was to Michigan's MS in biostats, not its PhD. In that case, North Carolina seems like a pretty clear choice. I don't go to there, but I am in the Triangle area and there are many good paying data science jobs, partially due to the presence of Duke, UNC, and NC State in the area. I believe also that UNC is supposed to have some people working on ML types of problems (Kosorok is an example of this). A friend of mine is in the program and she says there has been a strong push into data science type of coursework recently, if that is of interest. And for what its worth, North Carolina is a really nice place to live in my opinion. A bit of every season, mountains in the west and the beach to the east.
- 8 replies
-
- biostatistcs
- biostat
-
(and 3 more)
Tagged with:
-
Xiangning reacted to a post in a topic: Uchicago or UW stats master?
-
Perhaps you would clarify, as you referring to University of Washington or the University of Wisconsin? I think @bayessays above might've been referring to Washington with the Chicago being cheaper (although please correct me if I am wrong @bayessays!), whereas the reverse might be true of Washington. It also depends how quickly you finish these (Chicago's can be 1-2 years, but the few people I know who've done it have taken 2 years. I would also add that, in terms of rigor, most of the top MS programs seem relatively similar in terms of the required courses, so how hard you make it is somewhat dependent upon which courses you choose and if you are involved in extensive research.
- 13 replies
-
- statistics phd
- data scientist
- (and 3 more)
-
ENE1 reacted to a post in a topic: Fall 2020 Statistics Applicant Thread
-
Not to be confrontational, but if you're nearly as argumentative in person as with this internet persona, it might be hard developing the strong connections with your fellow PhD students that are often really beneficial to having a positive PhD experience. You have a terrific sounding background and got in to some really great schools so major props to you on that, but as a current PhD student in one of those schools I'll say that the confrontational style is probably best toned down a tad! We're all going through this wild, challenging experience, and I think helping each other out and building each other up is a good perspective to have. ? To the poster asking about Duke's admission chances, I do know that they *plan* on having another wave of acceptances. This past class was very large compared to their expectations, so I wonder if there is concern about having too large of a class this go around. Anywho good luck to all y'all that are waiting to hear back!
-
I would say that the courses are rigorous, but that there are very few requirements. Until the last year or so, technically there were no class requirements (although most students still took a majority of them). The qualifying exam after the first year is based on STA 711, STA 721, STA 611, STA 831, and STA 732. The courses tend to be decently rigorous. STA 711, STA 601, and STA 721 are taken in the Fall. STA 732, STA 831, and a case studies course are taken in the spring. STA 711 is a normal 1 semester measure-theoretic probability class (using A Probability Path). Here is the most recent course website: https://www2.stat.duke.edu/courses/Fall19/sta711/ STA 601 is an introduction to Bayesian Statistics, though it is better if some Bayesian Statistics is already known. It follow's Peter Hoff's book and is taught by Alexander Volfovsky. STA 721 is a theory based linear models class (mostly based off of Plane Answers to Complex Questions) that has a strong Bayesian emphasis. Here is the most recent class website: https://www2.stat.duke.edu/courses/Fall19/sta721/ STA 732 is the only "frequentist" theory course. Classical statistical theory is definitely minimally developed in the curriculum. STA 831 is a follow-up course to STA 601 and is purely Bayesian. There is no textbook the class follows. So the courses are difficult enough, but many students don't learn much non-Bayesian statistics, and some basic topics such as study design or generalized linear models are almost completely ignored (and neglected often in the elective offerings). Students going to Harvard or Washington (I know someone going to the latter) are taught far more classical statistics topics but don't have as much formal Bayesian coursework.
-
az25340 reacted to a post in a topic: Statistics PhD Application Evaluation
-
@Stat PhD Now Postdoc I can confirm that what you have said about Duke is at least somewhat true. Over the past 2 years, almost every admitted (matriculated, that is) student was a math major. However, it was frequently paired with another major (think economics, psychology), and the department seems to value that educational diversity. For what it is worth, the few students without as significant of a math background found the first year coursework exceedingly challenging. It has become the case that students with less mathematical maturity take some of the master's classes (for example, master's inference instead of PhD inference or delaying Measure Theory for a year), which delays the qualifying exams until the following year. I think there also is an associated challenge that, from my observations, professors tend to prefer the more mathematically inclined students, so those without such a background often have less advising options (although definitely still some!) available. That's just something to think about...