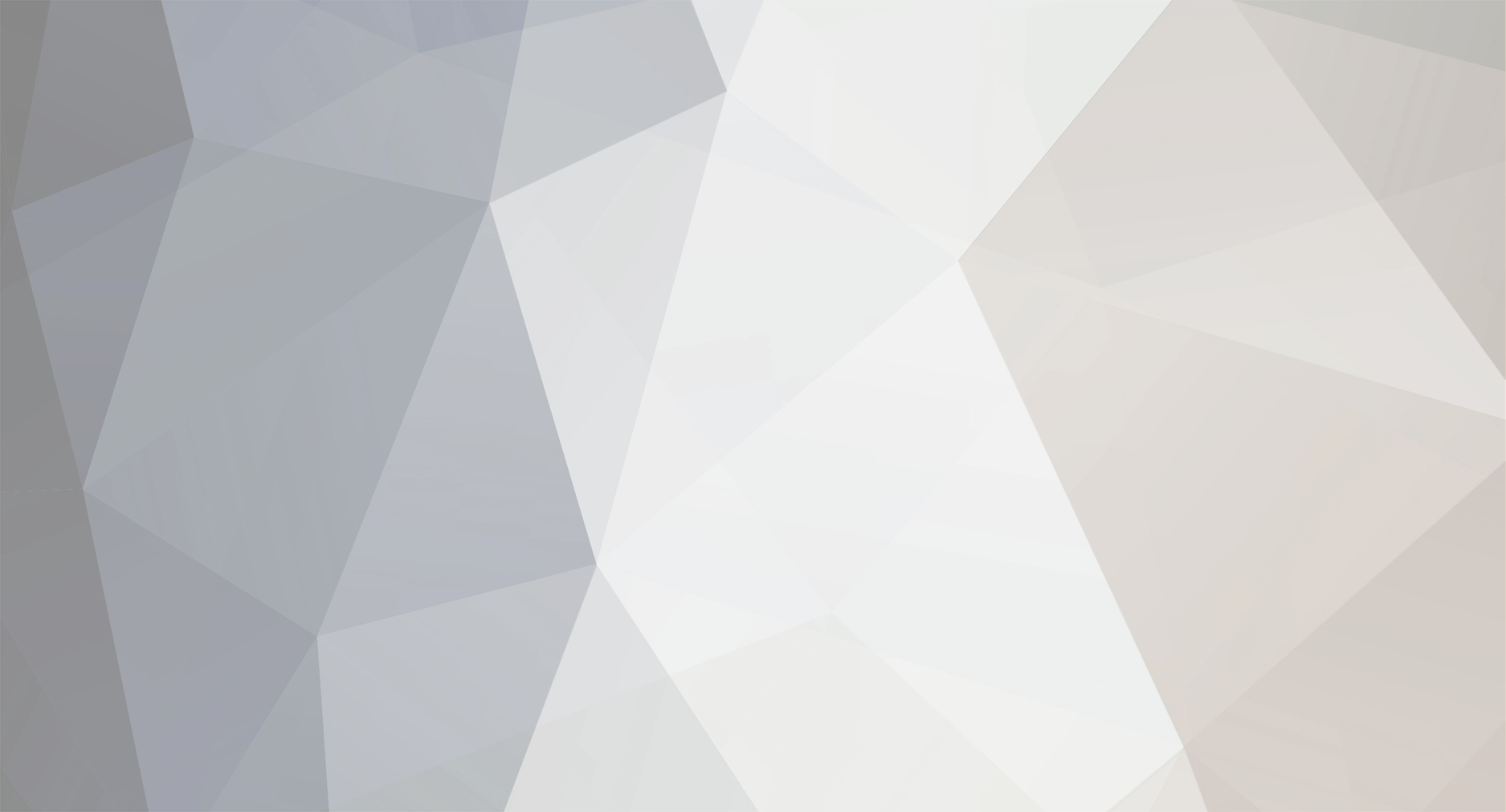
trynagetby
Members-
Posts
106 -
Joined
-
Last visited
Everything posted by trynagetby
-
Rankings before the Biostat/Stat separation
trynagetby replied to boulderV0's topic in Mathematics and Statistics
I think the point of the separation is that Biostatistics and Statistics programs are pretty different worlds and the comparison between departments in the two fields was not particularly productive.- 2 replies
-
- statistics phd
- biostatisticsphd
-
(and 2 more)
Tagged with:
-
Current Duke PhD student here. Last year at least 2 people got off the waitlist.
-
Is grad school still possible?
trynagetby replied to stars7000's topic in Mathematics and Statistics
applied statistics masters programs are not very competitive. If you get a good GRE score you can get into a few applied statistics professional/terminal masters. You might not be able to get into Stanford MS&Engineering, but you can definitely get into a few decent ones.- 4 replies
-
- mathematics
- finance
-
(and 2 more)
Tagged with:
-
Harvard MS Data Science vs Duke PhD Statistics
trynagetby replied to stackleberg cat's topic in Mathematics and Statistics
If you're worried about Duke being too Bayesian, I'd consider the fact that Duke's becoming significantly less Bayesian recently. Fan Li does causal inference research that's pretty non-bayesian, Alex Volfovsky works with Cynthia Rudin in the CS department on pretty straight up ML research, Jason Xu does mainly ML, Eric Laber (Susan Murhpy's student) does Susan Murphy type stuff. So if you're not a hardcore Bayesian there's still a lot of flexibility. -
Fall 2023 Stats PhD Profile Review
trynagetby replied to cmp024's topic in Mathematics and Statistics
I think your list is good with CMU will be the toughest nut to crack there. If you do well in Real Analysis, I think you'd be a serious consideration anywhere UW and below on the U.S News rankings (not to say you shouldn't apply higher, esp given diversity considerations). Also if you're seriously interested in Bayesian stuff, the obvious question is why not apply to Duke/UT Austin?- 2 replies
-
- statistics
- admissions
-
(and 2 more)
Tagged with:
-
Relative number of applicants by program type?
trynagetby replied to Cophysneurec's topic in Mathematics and Statistics
If you look at the number of applicants for schools that release data for previous years (Duke, Cornell for example) the number of applicants in the 2021-2022 admission cycle was very much within normal variation. So if the beginning of the pandemic didn't budge numbers too much, I don't think that this years numbers will depart from previous years very much either (aka there should not be a flood of applicants this year). Anecdotally while there are people who delayed applications because of Covid, in my experience there are also people moved their application up a year for various reasons (I did as well as some other people I know). So it's kind of a wash. -
Fall 2023 statistics/biostat PhD profile evaluation
trynagetby replied to rogarr's topic in Mathematics and Statistics
I think biology courses for biostat is borderline completely irrelevant. With regards to going into non-bio-related fields I don't think it matters that much if you plan it right. To be a finance quant at like a prop shot (Jane Street/Optiver/SIG) they literally only care about how well you ace the hard probability quant interviews. For other finance positions the name brand of the institution matters more. But I think doing research in related field (time series, stochastic procceses, applied probability) does help a lot, which could be slightly more difficult at a biostat department. For tech/institutional research it depends on what you research. If you're interested in causal inference and want to do that in industry biostat programs should be great to get top industry research jobs. But if you end up resarching very niche methods for flow cytometry or phylogentics or something it'll be difficult to get anything beyond your generic data science job at a tech company (which still pays lucratively ofc). Ofc if you're researching niche methods for flow cytometry or phylogentics you'd likely be working at an actual pharma/biotech company lol -
Fall 2023 statistics/biostat PhD profile evaluation
trynagetby replied to rogarr's topic in Mathematics and Statistics
If the recommender writer was on the admission committee for Wharton Stat I'd listen to him. But if he was on the admission committee for Penn finance I'd stick with the prevailing wisdom that Analysis I is probably the first grade that Stat AdCom look for on the transcript. I've seen people with diversity demographics or nontraditional/funky profiles get by with an eh grade with Real Analysis (or if they're EE or Physics Undergrad) but you definitely don't fall into that category. Good recommendations will go very far tho. I'd definitely encourage you to apply all the schools you'd want to go to if you can afford them, you never know what could happen. I definitely slightly regret not applying to enough *reach schools during my cycle. If you're legitimately interested in Biostat, I'd apply to like all the top ones where you think you'd be happy because I think you have a chance at all of them. Harvard, JHU, UW will be hard ofc but it wouldn't be impossible. The other good schools would be like Columbia, UNC, Umich, Penn, Duke etc... -
Fall 2023 statistics/biostat PhD profile evaluation
trynagetby replied to rogarr's topic in Mathematics and Statistics
Those schools are probably going to be VERY difficult. Still recommend applying to them if your rec writers are okay with them and you have the money. Additionally the jump from the Chicago/CMU/Wharton/UWashington/Columbia competitiveness tier to penn biostat tier is pretty dramatic. I'd add in schools like NCSU, UCLA, Winsconsin, UNC Biostat, Mich Biostat. IMO you have a much better shot at top Biostat programs than Stat programs given your good performance in stat classes. Also important note is if the graduate classes you mention are PhD or Masters. Masters graduate statistics classes are fairly meaningless in stat PhD admissions (as in they will not alleviate at all lack of advanced math). PhD graduate classes are a big value add (in that they can alleviate lack of advanced math). You should check out my profile to get an upperbound of what would be realistic. I was dinged at CMU and Waitlisted at UT Austin despite having more math. Unfortunately as an International Asian Male you're in the most competitive possible demographic category. If you don't get at least an A- in real analysis Wharton, CMU, Yale, Columbia, and Chicago are likely out of reach. If you're feeling good about your calculus a 80%+ on the Math subject test GRE would be extremely helpful. -
Profile Review - Statistics PhD
trynagetby replied to stackleberg cat's topic in Mathematics and Statistics
If you're domestic and not strapped for cash I'd advise adding schools on the upper end of rankings if you're interested in their programs (think Uwashington, Duke, etc...). This advice especially applies if you're very close with those two professors and expect good LOR. Edit: Not sure when all the application deadlines are so apologies if they have already passed. -
Profile eval fall 2023 PhD Biostat
trynagetby replied to strawberrychocolate's topic in Mathematics and Statistics
There's nothing wrong with an LAC! I think your list is still reasonable , if pretty top heavy. The PhD courses definitely help a lot and I think that's a reason why you have a good shot at those programs, but generally the rule is the more math you have as an undergrad the better. Honestly, the biggest factor will be the strength of your recommendation letters. If you think your letters are top-notch your list is probably good. Take my advice with a grain of salt as I only applied to 2 biostat schools, and got into 1.- 3 replies
-
- biostatistics
- statistics
-
(and 2 more)
Tagged with:
-
Profile eval fall 2023 PhD Biostat
trynagetby replied to strawberrychocolate's topic in Mathematics and Statistics
If you've had a lot of advanced mathematics background (Real Analysis I, II, Proof-Based Lin-Alg, + one more advanced class like Analysis of Algs, Abstract Algebra, Numerical analysis etc.. ) those schools are definitely all well within reach. If you've just had Real Analysis or even no Real Analysis you have a dece chance at all those schools but a clean reject from all of them is very well in the realm of possibility (I don't know anything about BU). Given your locational preference those schools pretty much cover the northeast. I'd throw in JHU too if Maryland isn't too south for you. Safe(r) schools would be like UNC, Umich, Duke. But those are pretty out your geographical preference.- 3 replies
-
- biostatistics
- statistics
-
(and 2 more)
Tagged with:
-
If you got A's in Real Analysis/Applied Analysis (which I'm guessing is like baby functional analysis?) I think you should apply to the range of NCSU/Wisconsin Madison/UIUC/Rice/Texas A&M and below. NCSU/Wisconsin might be a little of a reach but its pretty possible :). I'd also think about applying to programs like Umichigans Biostat Masters (which is funded) to give yourself a leg-up before going PhD. If Biostats is an option, UNC biostats and below should be a good chance. Good Luck!
-
PhD in Stats/Actuarial/Machine Learning
trynagetby replied to Ro2292's topic in Mathematics and Statistics
To be honest, I think applying to Finance Departments/Business schools/Operations Research will be better of a fit. -
I think you're tremendously underselling yourself. That's a whole lot of math, and not having life sciences coursework doesn't matter at all (I got an offer from Harvard Biostat and I had zero sciences background). Biostat at UW-Harvard-JHU might be too tough to crack, but I think an application to like UNC-Michigan would be worth it and I think you are very competitive at a place like Minnesota. For Statistics onwards I'd apply to a few schools at the level of Duke-UW-Michigan then work your way down to some safeties with the bulk of your applications in the USNR 20-40 range (I think Rice is a really safety school). Good luck!
-
Check out my profile from way back which I think is similar to yours but less OR focused. I also applied having no idea what OR was like and I got into Northwestern and GaTech ML (ISYE) with special fellowships for both. I only applied to the two because my main interests was Statistics PhDs. I think you have a good shot at all of them except MIT because, well, MIT (still worth applying IMO tho)
-
Best PhD programs for Causal Inference
trynagetby replied to forthorn's topic in Mathematics and Statistics
Harvard, Berkley, UW Stats all have at least 3 very top/rising star type people doing causal inference research. An important distinction you have to make is whether you want to do "classical" causal inference (propensity scores, average treatment effects, instrumental variables, potential outcomes framework) or "modern" causal inference (dags,judea pearl causal discovery, reinforcement learning, adaptive designs etc...). Both are pretty hot right now but the flavor of research is extremely different. -
Actuary looking to apply for statistics PhD
trynagetby replied to catarctica's topic in Mathematics and Statistics
If the OP has taken the equivalent of Math 55 he/she should definitely go for it. Harvard's the odd case as their undergraduate pure math first year math class literally has its own wikipedia page lol https://en.wikipedia.org/wiki/Math_55 -
Letter of recommendation question
trynagetby replied to Aspiring_stats_student2312's topic in Mathematics and Statistics
I'd say depends on the professor/class. If your Real Analysis class was super basic and your Complex Analysis class was like Stein and Shakarchi or Papa Rudin, I'd pick Complex Analysis. But if they're similar and you got into a competitive REU, you know apriori that the rec has to be somewhat decent right? -
I'd recommend watching this NIPs lecture from Robert Tibirishani https://www.microsoft.com/en-us/research/video/invited-talk-post-selection-inference-for-forward-stepwise-regression-lasso-and-other-procedures/?from=http%3A%2F%2Fresearch.microsoft.com%2Fapps%2Fvideo%2F%3Fid%3D259617 to get an idea of the type of problem that Statisticians are interested in and the inference/prediction ML distinction. It just seems you're not interested in statistical inference. There's nothing outdated about inference. It's just a goal that the statistics field is interested in, and the tools to achieve that tool are always evolving. I was a fairly mathematically/computationally mature undergraduate working with a super nice prof who works with undegrads. That type of program does seem well suited to your interests. But UCSD ECE is an extremely hard department to get into (as well as as NYU DS). You should still apply but hedge your bets with applications to bioinformatics/OR/BME programs as well.
-
Idk man, if you think you can get what you want out of a Statistics PhD then go for it. But it really doesn't sound like you'd enjoy the curriculum or research focus. Im quoting the first line out of the syllabus for the last course in Stanfords Statistical Inference Sequence: Testing problems in high dimensions: sparse alternatives (needle in a haystack) and nonsparse alternatives, Bonferroni's method, Fisher's test, ANOVA, higher criticism. Even CMU which is really MLey out of all the statistics departments requires to review topics like simple linear regression, ordinary least squares and weighted least squares, the geometry of least squares, quadratic forms, F tests and ANOVA tables, interval estimation, minimax theory, hypothesis testing, data reduction, convergence concepts You are interested in ML, but maybe not from a statistical perspective. Statisticians do all the things you're talking about but you absolutely have to prove inferential properties and understanding the basic foundations of hypothesis testing is necessary. Honestly, you should check out programs like https://bioinformatics.gatech.edu/ through the ISYE (read:OR). I think the problem here is "using high level programming languages/frameworks like Julia's Flux or the TF/PyTorch frameworks." When you're doing academic research you can't be constrained to pre-packaged stuff that everyone has access to. You have to do something novel and new to data which no one has before. That will inevitably involve implementing something from scratch. For example in my computational neuroscience research for a statistics prof at a top school, I once had to find the cluster of vectors lying on a sphere that maximized the sum of projection onto them by a given vector with certain contraints. How do you go about this the fastest, what data structure do you use, can you approximate? DS professors will want to know you have the tools to think about this. It's difficult to say what you should take. Analysis of Algorithms will be useful for jobs and might be enough to get your foot in the door some CS/OR places. Analysis I will be the only way you get into decent Statistics/Bio-statistics programs. Honestly I think you need to read dissertations from places like UWashington/Harvard/JHU biostat and really make sure you're not interested. You seem to be really hung up on hypothesis testing and asymptotics being boring when the concepts are kinda the core of Statistics.
-
Both would be important especially for NYU datascience, especially a formal AoA class (Dynamic programming, Graph Algorithms, basic NP-Completeness proofs) because professors need to know that you can reason rigorously about the complexity and correctness of novel algorithms. It seems like you're more interested in developing bioinformatic pipelines that use ML techniques than developing specific ML methods (at least in statistical sense). In modern statistics, optimization and modern techniques are important, but ultimately you have to prove that these techniques give good inference. Focus on modeling, inference and estimation are what differentiates Stats from other fields. While there are people in Stats who do the work with NN/VAEs/GAMs that you're talking about, they're the exception to the rule. People like Liam Paninski and John Cunningham from Columbia who do that type of stuff in statistics departments do so mainly in service to a field like neuroscience and I'm not really sure why their primary appointment is in the statistics department. I think you should seriously think about a PhD in like EE/Bioinformatics/Bioengineering/Computational Neuroscience if you're really not interested in inference and estimation. Idk what department you went to, but Stats PhD departments from Stanford to Berkley to Uchicago to Duke to Washington all require students to take heavy course load in classical inference (linear models, hypothesis testing, ANOVA) and asymptotic statistics. Albeit, the top departments like Stanford, CMU, Chicago, and Berkely put a very high dimensional spin on this, but its fundamentally the same goal. Correct me if I'm wrong, but I think all those departments are fairly modern. If every department is teaching these courses, I would think that they're fundamental to statistics. Lower ranked programs are even more focused on "classical statistics". If you attend these programs without interest in inference, you'll at least be bored for a year. Also take my rambling with a grain of salt, I'm an incoming grad student and I don't even hold a masters.