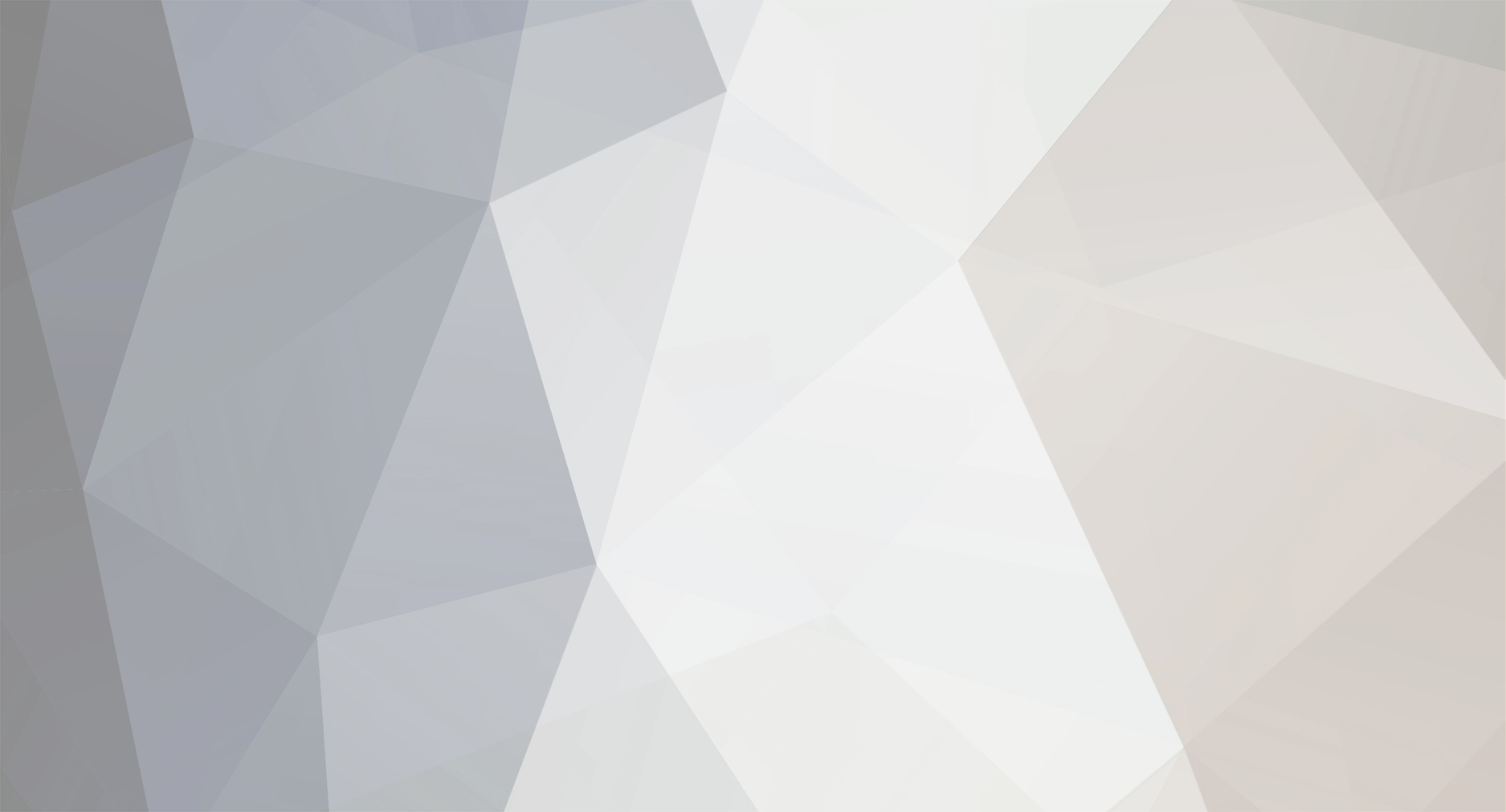
icantdoalgebra
Members-
Posts
43 -
Joined
-
Last visited
icantdoalgebra's Achievements
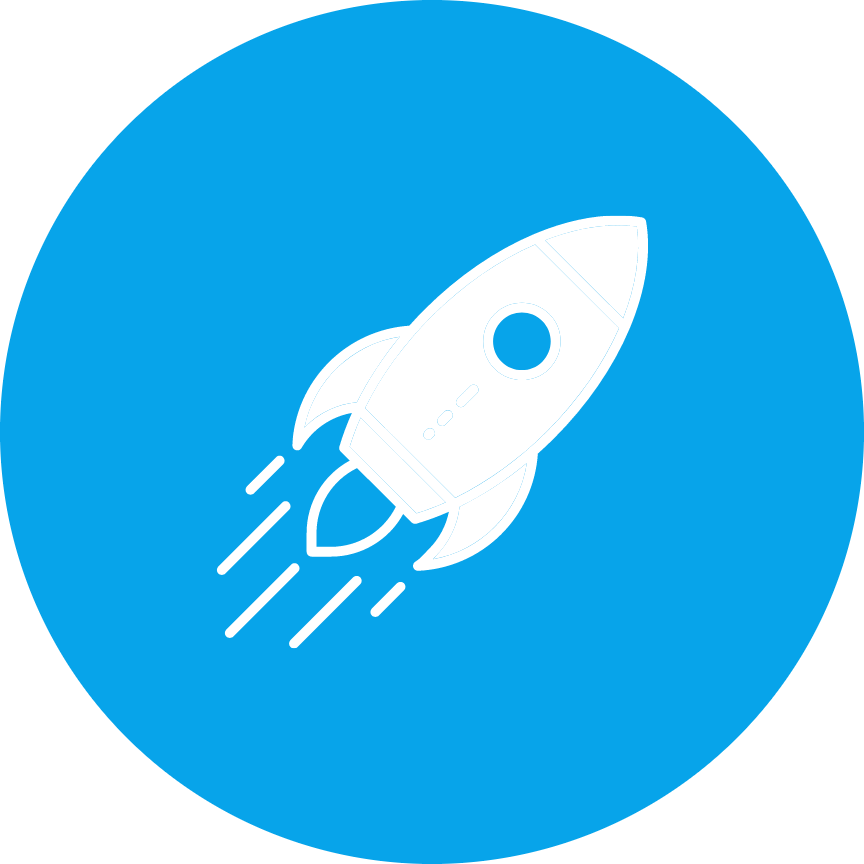
Caffeinated (3/10)
25
Reputation
-
gouda91 reacted to a post in a topic: Top Stat PhD programs 2021
-
icantdoalgebra reacted to a post in a topic: Virtual Panel on Statistics PhD Programs
-
liyu reacted to a post in a topic: School suggestions?
-
MLE reacted to a post in a topic: Fall 2021 Statistics/Biostatistics Applicant Thread
-
Last year I've asked for an extension on April 15th due to getting an offer very late in the cycle. IMO it doesn't hurt to try and ask at least; the most likely worst-case scenario is that your request for extension gets denied (I would be quite shocked if anything worse than this happens) but there is a chance that they would grant you an extension.
-
icantdoalgebra reacted to a post in a topic: Fall 2021 Statistics/Biostatistics Applicant Thread
-
statsnow reacted to a post in a topic: Biostats PhD/Masters 2021: Profile Eval
-
Biostats PhD/Masters 2021: Profile Eval
icantdoalgebra replied to cctvwp's topic in Mathematics and Statistics
As a side note to this point, a lot of Berkeley's most famous statisticians have passed away. Note this is not particularly relevant to the current discussion at hand since we are discussing the departments today (and also because ... dead people don't do research), but if we do want to talk about "revolutionary" developments I think it is somewhat worthwhile to bring up Berkeley's significance (although it really shouldn't affect anybody's decision in where to go or departmental rankings because this is quite historical). Jerzy Neyman was a professor at Berkeley and was the key driving force behind the formation of the department and he was no slouch when it came to revolutionary developments (quotes deliberately omitted because confidence intervals, hypothesis testing, potential outcomes are all, beyond any doubt, revolutionary developments). George Dantzig was a student and professor at Berkeley for a while (although he left for Stanford later) but while at Berkeley he developed the simplex algorithm which may not be revolutionary in statistics, but is the foundation for several other fields. David Blackwell was a professor at Berkeley for several decades; you might know him from Rao-Blackwell, but he also did a lot more than that (Blackwell-Macqueen, etc.). There are a few others that can be mentioned too, David Freedman has had quite a bit of influence outside the world of statistics (and in statistical education), Leo Breiman has been mentioned above (CART and random forests), Le Cam and his work on lower bounds, and several more. I bring them up not because I'm trying to argue against any of the positions taken here: I agree that Stanford's statistics department is better today. It is in a class of its own when you consider traditional statistics. But I still feel somewhat obligated to defend Berkeley if we are mentioning "revolutionary" developments without mentioning Berkeley's own rich history. However let me re-emphasize, none of the above should be a part of one's consideration for picking a program for grad school nor should it be a part of decision making about which departments are stronger. -
icantdoalgebra reacted to a post in a topic: Please Advise: Stanford vs Berkeley
-
statsnow reacted to a post in a topic: Top Stat PhD programs 2021
-
Easy; Peter Bickel, Michael Jordan, Martin Wainwright. People like van der Laan, Bartlett, Brillinger, Aldous, Yu, and Pitman might not be as famous as those Stanford faculty that you listed, but its not like they are some random professors. How about Fernando Perez and his work on creating Jupyter Notebooks? Perhaps its not as much of a research accomplishment, but creating a widely-used software that makes performing statistics and data science easier is definitely worth quite a bit of influence. I'm not trying to argue that Berkeley is better, in fact I agree that Stanford's program is better. However is it substantially better? I am skeptical. There is a valid point though, in that Berkeley's stats department has leaned more heavily towards machine learning recently and if you are a statistics purist you could reasonably make the argument that Stanford is substantially better if that is your criteria for evaluation.
-
MLE reacted to a post in a topic: Affirmative action in admissions and supporting students of diverse backgrounds
-
frequentist reacted to a post in a topic: Affirmative action in admissions and supporting students of diverse backgrounds
-
stemstudent12345 reacted to a post in a topic: Affirmative action in admissions and supporting students of diverse backgrounds
-
If any stats program decided to do this, you would instantly know to avoid it because this is, by definition, extremely horrible statistics. The goal of an admissions committee is to try and pick the candidates that has the highest future success (of which is measured to whatever the committee decides to chose) subject to a series of resource constraints. Ultimately there is a regression problem involved: trying to predict future outcomes given the applicant profile. You are proposing for the committee to throw out all covariates that may have predictive power on the outcome and replace it with a single measured value (the proposed test score), whereas any statistician with half a brain would propose the exact opposite. The admissions committee may not be running a linear regression or some ML algorithm to try and predict what will happen to candidates in the future, but the point still stands. Having more than just one feature to look at is often superior to only looking at one feature and trying to predict with that.
-
icantdoalgebra reacted to a post in a topic: Choosing Statistics PhD: Harvard vs Berkeley?
-
Having a award itself doesn't mean much, but usually stronger candidates tend to have more awards. As an aside, you should just be focusing on doing good research with your current professor; all the professors in the stats department at Berkeley are highly qualified (that's what it means to be a top department) so even if they aren't Lebron James, a strong letter of recommendation from them will carry a lot of weight amongst admission committees. Fixating on the fame of the professor might turn out to be quite counter-productive to your current research. As per your point of delaying graduation, I really don't know what the optimal move is. I don't think having good grades in 210A/B will be that much more valuable than just having taken 210A because the grading in grad classes is quite generous. Having another research opportunity to replace a recommendation letter which basically states you did well in a class would be helpful but is it worth delaying graduation for? I have no clue.
-
MLE reacted to a post in a topic: Fall 2021 Statistics/Biostatistics Applicant Thread
-
listar2000 started following icantdoalgebra
-
You should be a strong candidate for a place like Duke, but for Berkeley and Stanford I'd say you have a decent chance but I wouldn't bet on it (but I wouldn't bet against though). I was class of 2020 at Cal and it seems like your profile is somewhat weaker than some of my friends, both in terms of coursework and research; none of us got into Stanford or (I think) Columbia, and very few of us got into Berkeley. However this assessment is based on your current position, and a lot can change in the span of a year. As for your question about research publications, I'd say the actual research you do matters more than any publication. People with publications tend to better in admissions not because of publications, but because usually those with publications have stronger relationships with the professors writing them recommendation letters. I had zero publications when applying to grad school and I got into places, as did some of my friends. About delaying applying to a year later, I had asked essentially the same question to a lot of people, and the response I got was mostly "it really doesn't matter". It doesn't seem like they have higher requirements for those who apply the year after graduating but its not like you can do much to strengthen your application further with the exception of finding another research opportunity.
-
icantdoalgebra reacted to a post in a topic: TIFU on my CV
-
icantdoalgebra reacted to a post in a topic: How much do Stats PhD receive in funding per year?
-
MLE reacted to a post in a topic: I did not take Multivariable calculus but Analysis I. Do I still need to take Multivariable calculus? (Ph.D. applicant)
-
I didn't take multivariable calculus when applying last year and didn't have trouble getting into programs. I think having more advanced classes should make up for not having an intro class like multivariate, but that's just my guess and maybe you should take it to be on the safe side? I'm not too certain what the correct move is.
-
Non-Responsive Letter Writer/ Late Letters
icantdoalgebra replied to bob loblaw's topic in Mathematics and Statistics
So most of the programs I applied to allows for late submission of letters, especially those with December 1st deadlines. As for whether it has an impact or not, it's hard to say. I've had a professor tell me that if it comes late for December 1st deadlines it doesn't really matter because nobody wants to look at applications seriously before the New Year. As for personal anecdotes: my rec letter came in late for the early December deadlines, and I got into one out of three programs but this is very low signal information since its a sample size of N=3 and the difficulty of admission is quite different in this group. -
Isn't that more due to the fact that MIT primarily only has people in either ML or high-dimensional statistics (and in this case I feel like the opportunity of working with Candes at Stanford or Wainwright at Berkeley is probably better than being at MIT), but there's more to statistics than these two fields: what if the person wanted to do something in causal inference or post-selective inference? MIT would clearly be not as great compared to other top stats programs. Additionally what makes a good EECS application is pretty different from what makes a good stats application: applying to EECS in ML usually revolves around having published somewhere in ICML or NeurIPS (sometimes even multiple times) as an undergrad, and comparatively grades/coursework really don't matter that much. Perhaps this is true in general about stats program curricula being outdated but if we are comparing Stanford/Berkeley to MIT this isn't really that true nowadays. People in the stat department who are interested in ML are encouraged to go take courses in optimization and information theory (which is what I am doing) because as you said, they are very useful. In fact one of the core courses at Berkeley gives a brief introduction to information theory because of how useful it is. But again, not everybody wants to do ML/data science/what-ever gets hyped up in the media nowadays and places like MIT EECS/Math or Princeton ORFE are really niche recommendations since they tend to lean very heavily towards a small subset of areas within statistics and have virtually no presence elsewhere. Also about your point for professorship, I know Berkeley gave out 3 offers for faculty last year, and 2 of them are for somebody with a traditional statistics background. @kimmy sorry for the sidetracking but the people on this forum are pretty good at giving advice on applying to stats programs (probably because there are a few stats professors running around on these forums). I'd be somewhat more skeptical of any advice you'd receive here about applying to math or EECS (as to the best of my knowledge) because there aren't any similar such people on this forum and you should probably go look elsewhere for advice but do consider these options if that is something you are interested about.
-
Pure Math PhD Profile Evaluation
icantdoalgebra replied to thorinandco's topic in Mathematics and Statistics
As recommended go check out the mathematicsgre forum, there's a lot more people there involved in math. But I will say that I know many people with applications that are quite a bit stronger than yours (people at schools like Berkeley, Stanford, and Harvard) with higher gpas, 5+ graduate courses (some even reaching double digits!), multiple high-quality REUs, GRE scores in the 90+ percentiles who weren't able to crack programs like Princeton. Your recommendations seems like a bit of a wildcard but I can imagine a situation where if your profs call you brilliant in the rec letters you may get in. If you have some sort of story about overcoming adversity with your low CC GPA and turning it around when you transferred, manage to get a pretty high score on the subject test I don't think Berkeley is an impossibility, but still a reasonable reach. You should take all my advice with a grain of salt though as math is not my area.- 2 replies
-
- math
- evaluation
-
(and 2 more)
Tagged with:
-
Profile Evaluation for Fall 20201 Biostat PhD/MS
icantdoalgebra replied to tukey's topic in Mathematics and Statistics
I think you should clarify if its a school known for grade deflation or not. If its UChicago or Caltech the response might be different than if it were say Harvard or Yale. I would be overstepping my expertise if I tried to tell you what schools are a target vs reach but I could imagine the answers changing. Maybe others can comment on this perspective. -
Profile Evaluation For Stats Phd 2021 fall
icantdoalgebra replied to nauhark's topic in Mathematics and Statistics
You still have roughly 5-6 months before you need to apply; you can find a research opportunity in statistics at least by the time the fall semester starts, and doing a semester of stats research would get you a pretty strong rec letter. It shouldn't be that difficult to do considering your grades and the fact that you are at an Ivy league school. Usually domestic students don't have meaningful theoretical research (although this is becoming less and less true) before applying to a PhD program, but international students at the top programs often do (to be fair I've only interacted with those at Berkeley but I'd assume it be similar at peer institutions). If you think that taking a gap year means that you get to apply later and have an easier time getting in due to Covid being over, I'd say just apply this year; there's really no guarantee things will be better. If you can do something in the gap year that would greatly improve your application, like a serious theoretical statistics research opportunity, its not a bad idea. -
Laptop suggestions for math/statistics grad schools
icantdoalgebra replied to J456's topic in Mathematics and Statistics
I would second the Macbook if you aren't that tech-savvy, as having a Unix based OS is much easier to deal with than a Windows one for anything programming related. If you don't like Apple, then an option is to dual boot Linux on whatever Windows machine you buy. For anything that requires intensive compute, your university will likely either have a cluster or provide you with cloud compute credits, in which case you would need to ssh into and having a Unix OS makes this process significantly less painful. The Windows Linux support IMO is frankly quite awful and package management/installing new programming libraries is also horrendous on Windows. Also second the insanely expensive graphics card, think about how fast your ggplots will render with real time ray tracing.